All Publications
Explore our research publications. Use tags like Representation Learning (RepL) and Computational Social Science (CSS) to filter the papers.
- All
- CSS
- RepL
TakeLab Retriever: AI-Driven Search Engine for Articles from Croatian News Outlets
arXiv preprint
TakeLab Retriever is an AI-driven search engine designed to discover, collect, and semantically analyze news articles from Croatian news outlets. It offers a unique perspective on the history and current landscape of Croatian online news media, making it an essential tool for researchers seeking to uncover trends, patterns, and correlations that general-purpose search engines cannot provide. TakeLab retriever utilizes cutting-edge natural language processing (NLP) methods, enabling users to sift through articles using named entities, phrases, and topics through the web application. This technical report is divided into two parts: the first explains how TakeLab Retriever is utilized, while the second provides a detailed account of its design. In the second part, we also address the software engineering challenges involved and propose solutions for developing a microservice-based semantic search engine capable of handling over ten million news articles published over the past two decades.
Are ELECTRA’s Sentence Embeddings Beyond Repair? The Case of Semantic Textual Similarity
In Findings of the Association for Computational Linguistics: EMNLP 2024, pages 9159–9169, Miami, Florida, USA. Association for Computational Linguistics.
While BERT produces high-quality sentence embeddings, its pre-training computational cost is a significant drawback. In contrast, ELECTRA provides a cost-effective pre-training objective and downstream task performance improvements, but worse sentence embeddings. The community tacitly stopped utilizing ELECTRA’s sentence embeddings for semantic textual similarity (STS). We notice a significant drop in performance for the ELECTRA discriminator’s last layer in comparison to prior layers. We explore this drop and propose a way to repair the embeddings using a novel truncated model fine-tuning (TMFT) method. TMFT improves the Spearman correlation coefficient by over 8 points while increasing parameter efficiency on the STS Benchmark. We extend our analysis to various model sizes, languages, and two other tasks. Further, we discover the surprising efficacy of ELECTRA’s generator model, which performs on par with BERT, using significantly fewer parameters and a substantially smaller embedding size. Finally, we observe boosts by combining TMFT with word similarity or domain adaptive pre-training.
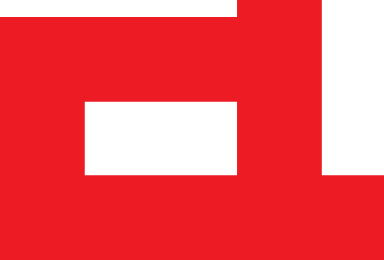
Closed-domain event extraction for hard news event monitoring: a systematic study
PeerJ Computer Science
News event monitoring systems allow real-time monitoring of a large number of events reported in the news, including the urgent and critical events comprising the so-called hard news. These systems heavily rely on natural language processing (NLP) to perform automatic event extraction at scale. While state-of-the-art event extraction models are readily available, integrating them into a news event monitoring system is not as straightforward as it seems due to practical issues related to model selection, robustness, and scale. To address this gap, we present a study on the practical use of event extraction models for news event monitoring. Our study focuses on the key task of closed-domain main event extraction (CDMEE), which aims to determine the type of the story’s main event and extract its arguments from the text. We evaluate a range of state-of-the-art NLP models for this task, including those based on pre-trained language models. Aiming at a more realistic evaluation than done in the literature, we introduce a new dataset manually labeled with event types and their arguments. Additionally, we assess the scalability of CDMEE models and analyze the trade-off between accuracy and inference speed. Our results give insights into the performance of state-of-the-art NLP models on the CDMEE task and provide recommendations for developing effective, robust, and scalable news event monitoring systems.
Looking Right is Sometimes Right: Investigating the Capabilities of Decoder-only LLMs for Sequence Labeling
In Findings of the Association for Computational Linguistics: ACL 2024, pages 14168–14181, Bangkok, Thailand. Association for Computational Linguistics.
Pre-trained language models based on masked language modeling (MLM) excel in natural language understanding (NLU) tasks. While fine-tuned MLM-based encoders consistently outperform causal language modeling decoders of comparable size, recent decoder-only large language models (LLMs) perform on par with smaller MLM-based encoders. Although their performance improves with scale, LLMs fall short of achieving state-of-the-art results in information extraction (IE) tasks, many of which are formulated as sequence labeling (SL). We hypothesize that LLMs’ poor SL performance stems from causal masking, which prevents the model from attending to tokens on the right of the current token. Yet, how exactly and to what extent LLMs’ performance on SL can be improved remains unclear. We explore techniques for improving the SL performance of open LLMs on IE tasks by applying layer-wise removal of the causal mask (CM) during LLM fine-tuning. This approach yields performance gains competitive with state-of-the-art SL models, matching or outperforming the results of CM removal from all blocks. Our findings hold for diverse SL tasks, demonstrating that open LLMs with layer-dependent CM removal outperform strong MLM-based encoders and even instruction-tuned LLMs.
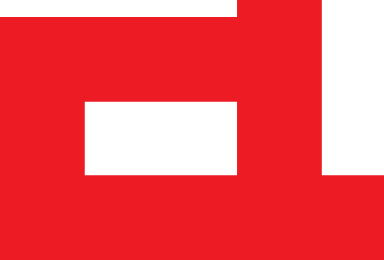
Disentangling Latent Shifts of In-Context Learning Through Self-Training
arXiv preprint
In-context learning (ICL) has become essential in natural language processing, particularly with autoregressive large language models capable of learning from demonstrations provided within the prompt. However, ICL faces challenges with stability and long contexts, especially as the number of demonstrations grows, leading to poor generalization and inefficient inference. To address these issues, we introduce STICL (Self-Training ICL), an approach that disentangles the latent shifts of demonstrations from the latent shift of the query through self-training. STICL employs a teacher model to generate pseudo-labels and trains a student model using these labels, encoded in an adapter module. The student model exhibits weak-to-strong generalization, progressively refining its predictions over time. Our empirical results show that STICL improves generalization and stability, consistently outperforming traditional ICL methods and other disentangling strategies across both in-domain and out-of-domain data.
Leveraging Open Information Extraction for More Robust Domain Transfer of Event Trigger Detection
In Findings of the Association for Computational Linguistics: EACL 2024, pages 1197–1213, St. Julian’s, Malta. Association for Computational Linguistics.
Event detection is a crucial information extraction task in many domains, such as Wikipedia or news. The task typically relies on trigger detection (TD) – identifying token spans in the text that evoke specific events. While the notion of triggers should ideally be universal across domains, domain transfer for TD from high- to low-resource domains results in significant performance drops. We address the problem of negative transfer in TD by coupling triggers between domains using subject-object relations obtained from a rule-based open information extraction (OIE) system. We demonstrate that OIE relations injected through multi-task training can act as mediators between triggers in different domains, enhancing zero- and few-shot TD domain transfer and reducing performance drops, in particular when transferring from a high-resource source domain (Wikipedia) to a low(er)-resource target domain (news). Additionally, we combine this improved transfer with masked language modeling on the target domain, observing further TD transfer gains. Finally, we demonstrate that the gains are robust to the choice of the OIE system.
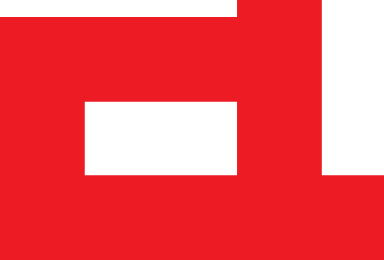
Parameter-Efficient Language Model Tuning with Active Learning in Low-Resource Settings
In Proceedings of the 2023 Conference on Empirical Methods in Natural Language Processing, pages 5061–5074, Singapore. Association for Computational Linguistics.
Pre-trained language models (PLMs) have ignited a surge in demand for effective fine-tuning techniques, particularly in low-resource domains and languages. Active learning (AL), a set of algorithms designed to decrease labeling costs by minimizing label complexity, has shown promise in confronting the labeling bottleneck. In parallel, adapter modules designed for parameter-efficient fine-tuning (PEFT) have demonstrated notable potential in low-resource settings. However, the interplay between AL and adapter-based PEFT remains unexplored. We present an empirical study of PEFT behavior with AL in low-resource settings for text classification tasks. Our findings affirm the superiority of PEFT over full-fine tuning (FFT) in low-resource settings and demonstrate that this advantage persists in AL setups. We further examine the properties of PEFT and FFT through the lens of forgetting dynamics and instance-level representations, where we find that PEFT yields more stable representations of early and middle layers compared to FFT. Our research underscores the synergistic potential of AL and PEFT in low-resource settings, paving the way for advancements in efficient and effective fine-tuning.
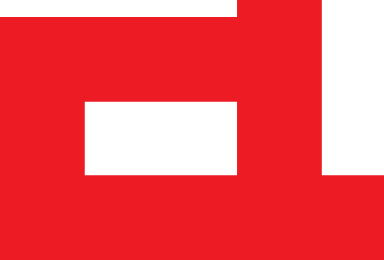
Smooth Sailing: Improving Active Learning for Pre-trained Language Models with Representation Smoothness Analysis
In Proceedings of the 2023 CLASP Conference on Learning with Small Data (LSD), pages 11–24, Gothenburg, Sweden. Association for Computational Linguistics.
Developed to alleviate prohibitive labeling costs, active learning (AL) methods aim to reduce label complexity in supervised learning. While recent work has demonstrated the benefit of using AL in combination with large pre-trained language models (PLMs), it has often overlooked the practical challenges that hinder the effectiveness of AL. We address these challenges by leveraging representation smoothness analysis to ensure AL is feasible, that is, both effective and practicable. Firstly, we propose an early stopping technique that does not require a validation set – often unavailable in realistic AL conditions – and observe significant improvements over random sampling across multiple datasets and AL methods. Further, we find that task adaptation improves AL, whereas standard short fine-tuning in AL does not provide improvements over random sampling. Our work demonstrates the usefulness of representation smoothness analysis for AL and introduces an AL stopping criterion that reduces label complexity.
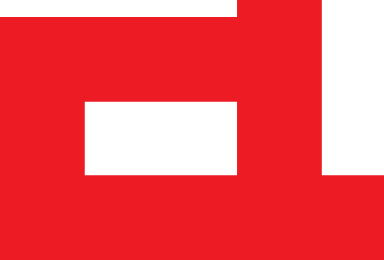
Easy to Decide, Hard to Agree: Reducing Disagreements Between Saliency Methods
In Findings of the Association for Computational Linguistics: ACL 2023, pages 9147–9162, Toronto, Canada. Association for Computational Linguistics.
A popular approach to unveiling the black box of neural NLP models is to leverage saliency methods, which assign scalar importance scores to each input component. A common practice for evaluating whether an interpretability method is faithful has been to use evaluation-by-agreement – if multiple methods agree on an explanation, its credibility increases. However, recent work has found that saliency methods exhibit weak rank correlations even when applied to the same model instance and advocated for alternative diagnostic methods. In our work, we demonstrate that rank correlation is not a good fit for evaluating agreement and argue that Pearson-r is a better-suited alternative. We further show that regularization techniques that increase faithfulness of attention explanations also increase agreement between saliency methods. By connecting our findings to instance categories based on training dynamics, we show that the agreement of saliency method explanations is very low for easy-to-learn instances. Finally, we connect the improvement in agreement across instance categories to local representation space statistics of instances, paving the way for work on analyzing which intrinsic model properties improve their predisposition to interpretability methods.
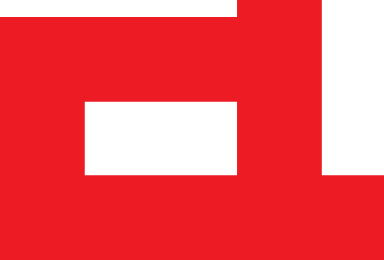
ALANNO: An Active Learning Annotation System for Mortals
In Proceedings of the 17th Conference of the European Chapter of the Association for Computational Linguistics: System Demonstrations, pages 228–235, Dubrovnik, Croatia. Association for Computational Linguistics.
Supervised machine learning has become the cornerstone of today’s data-driven society, increasing the need for labeled data. However, the process of acquiring labels is often expensive and tedious. One possible remedy is to use active learning (AL) – a special family of machine learning algorithms designed to reduce labeling costs. Although AL has been successful in practice, a number of practical challenges hinder its effectiveness and are often overlooked in existing AL annotation tools. To address these challenges, we developed ALANNO, an open-source annotation system for NLP tasks equipped with features to make AL effective in real-world annotation projects. ALANNO facilitates annotation management in a multi-annotator setup and supports a variety of AL methods and underlying models, which are easily configurable and extensible.
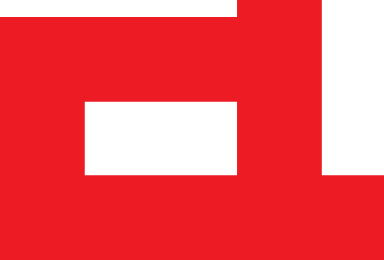
You Are What You Talk About: Inducing Evaluative Topics for Personality Analysis
Findings of the Association for Computational Linguistics: EMNLP 2022, pages 3986–3999, Abu Dhabi, United Arab Emirates. Association for Computational Linguistics.
Expressing attitude or stance toward entities and concepts is an integral part of human behavior and personality. Recently, evaluative language data has become more accessible with social media’s rapid growth, enabling large-scale opinion analysis. However, surprisingly little research examines the relationship between personality and evaluative language. To bridge this gap, we introduce the notion of evaluative topics, obtained by applying topic models to pre-filtered evaluative text from social media. We then link evaluative topics to individual text authors to build their evaluative profiles. We apply evaluative profiling to Reddit comments labeled with personality scores and conduct an exploratory study on the relationship between evaluative topics and Big Five personality facets, aiming for a more interpretable, facet-level analysis. Finally, we validate our approach by observing correlations consistent with prior research in personality psychology.
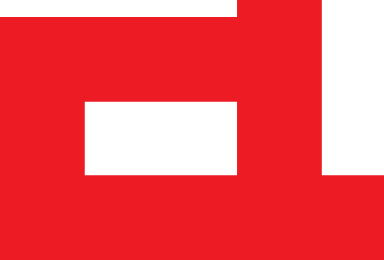